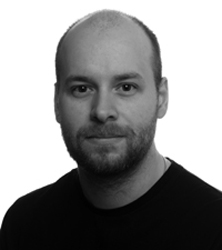
Predictive Maintenance (PdM) using Artificial Intelligence (AI) and Machine Learning (ML) is the top-ranked use case in terms of business value of industrial digitalization. Not surprising since the annual maintenance cost in Swedish manufacturing industry is over 100 billion SEK and 60% of all maintenance activities are reactive.
The PACA project, coordinated by Chalmers, aims to develop PdM algorithms, based on advanced cluster analysis, to increase the precision and make them understandable for decision makers.
Data from multiple streams
Three real-world cases provide data from multiple streams (sensors and computer systems) and multiple machines. The data will be jointly analysed to identify interesting patterns and compare across machines and their historical records. This will build understanding of how different patterns correlate to certain wear-down behaviour, later used to design an algorithm for prediction of future machine states/failures.
Improving Smart maintenance
Expected effects include: increased productivity, robustness, resource efficiency and competence in Smart Maintenance and advanced data analysis. The cross-disciplinary consortium consists of major manufacturing companies, service and IT providers, and universities with expertise in Smart Maintenance and advanced data science.